Great Health Great Fitness
“Impact of Artificial Intelligence on Health: Opportunities, Challenges, and Ethical Considerations”
Artificial intelligence (AI) is revolutionizing healthcare, offering immense opportunities to improve patient outcomes, enhance efficiency, and drive innovation. However, its implementation also brings forth significant challenges and ethical considerations that demand careful navigation. From personalized treatment recommendations to concerns about data privacy and algorithmic bias, the impact of AI on health is profound and multifaceted. Balancing the promise of AI with ethical principles and regulatory considerations is essential to ensure that AI serves the best interests of patients and promotes equitable, patient-centered care.
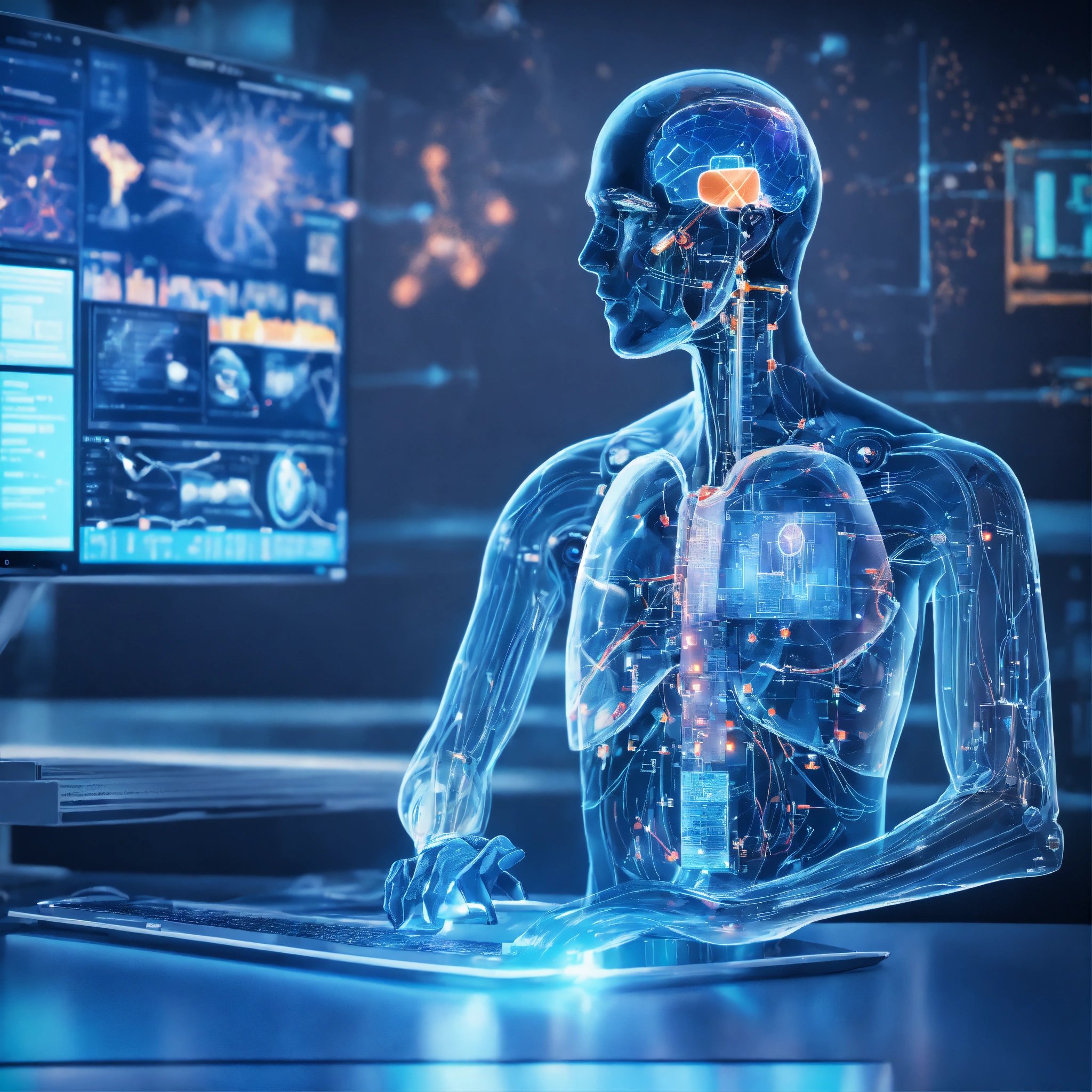
Introduction
Artificial intelligence (AI) has emerged as a transformative force in healthcare, offering unprecedented opportunities to revolutionize medical practice, research, and patient care. By harnessing the power of advanced algorithms, machine learning, and big data analytics, AI has the potential to enhance diagnosis, treatment, and healthcare delivery in ways previously unimaginable. However, along with its promise come significant challenges and ethical considerations that must be carefully navigated to realize the full potential of AI in health.
In this essay, we will explore the impact of artificial intelligence on health, examining the opportunities it presents, the challenges it poses, and the ethical considerations that arise from its implementation. Through a comprehensive analysis of current trends, case studies, and future prospects, we will delve into the multifaceted landscape of AI in healthcare, highlighting key issues and implications for patients, healthcare professionals, researchers, and policymakers alike.
As we embark on this exploration, it is crucial to recognize the transformative potential of AI in improving healthcare outcomes, advancing medical knowledge, and promoting population health. From personalized treatment regimens tailored to individual patient profiles to predictive analytics that forecast disease outbreaks and inform public health interventions, the possibilities are vast and far-reaching. Moreover, AI holds the promise of addressing longstanding challenges in healthcare, such as reducing diagnostic errors, optimizing resource allocation, and increasing access to care, particularly in underserved communities.
However, amidst the optimism surrounding AI’s potential lies a complex array of challenges and ethical dilemmas that demand careful consideration. Concerns about data privacy and security, algorithmic bias and fairness, regulatory oversight, and the societal implications of AI-driven healthcare systems loom large. Moreover, questions of patient autonomy, transparency, and accountability raise important ethical considerations that must be addressed to ensure that AI in serves the best interests of individuals and communities.
Opportunities of AI in Health
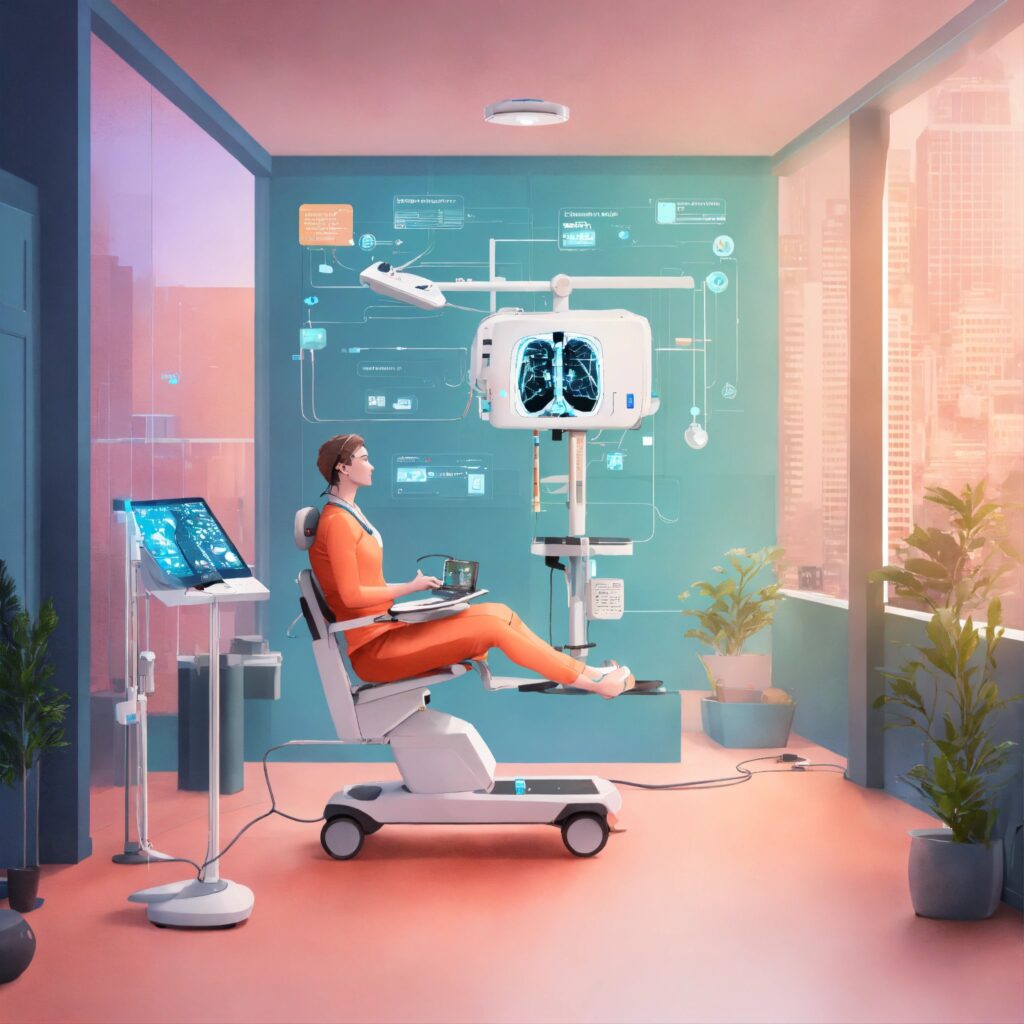
Artificial intelligence (AI) presents a multitude of opportunities to revolutionize healthcare across various domains. By harnessing the power of advanced algorithms and machine learning techniques, AI has the potential to significantly improve patient outcomes, enhance healthcare delivery, and accelerate medical innovation. Here are some of the key opportunities that AI offers in health:
- Improving Diagnosis and Disease Detection: AI-powered diagnostic tools can analyze medical images, such as X-rays, MRIs, and CT scans, with unparalleled accuracy and efficiency. Machine learning algorithms can learn from vast datasets of labeled images to detect subtle patterns and anomalies indicative of diseases, enabling earlier and more accurate diagnoses. For example, AI algorithms have shown promise in detecting early signs of diseases like cancer, cardiovascular conditions, and neurological disorders, leading to timely interventions and improved patient outcomes.
- Personalized Treatment and Precision Medicine: AI enables the analysis of large-scale genomic, clinical, and lifestyle data to tailor treatment plans to individual patient profiles. By identifying genetic markers, biomarkers, and other predictive factors, AI algorithms can help clinicians predict treatment responses, optimize drug dosages, and select the most effective therapies for each patient. Precision medicine approaches powered by AI hold the potential to revolutionize healthcare by delivering targeted interventions that maximize efficacy while minimizing adverse effects.
- Enhancing Healthcare Delivery and Efficiency: AI-driven technologies streamline administrative tasks, automate routine processes, and optimize resource allocation, leading to greater efficiency and cost-effectiveness in healthcare delivery. Natural language processing (NLP) algorithms can transcribe clinical notes, extract relevant information from medical records, and automate documentation tasks, freeing up clinicians’ time for patient care. Additionally, predictive analytics models can forecast patient demand, optimize hospital workflows, and reduce wait times, improving the overall patient experience and healthcare quality.
- Supporting Medical Research and Drug Discovery: AI accelerates the pace of medical research and drug development by analyzing vast amounts of biomedical data, identifying novel drug targets, and predicting drug interactions and side effects. Machine learning algorithms can analyze genomics data, protein structures, and drug chemical properties to uncover new insights into disease mechanisms and potential therapeutic interventions. AI-powered drug discovery platforms enable researchers to screen large compound libraries and identify promising candidates for further development, expediting the drug discovery process and bringing new treatments to market more rapidly.
- Remote Monitoring and Telemedicine: AI-enabled remote monitoring devices and telemedicine platforms empower patients to manage their health from the comfort of their homes while enabling healthcare providers to deliver timely interventions and personalized care remotely. Wearable devices equipped with AI algorithms can continuously monitor vital signs, detect changes in health status, and alert patients and caregivers to potential risks. Telemedicine platforms powered by AI facilitate virtual consultations, remote diagnosis, and remote patient monitoring, expanding access to healthcare services, particularly in rural and underserved areas.
Overall, the opportunities presented by AI are vast and transformative, offering the potential to improve patient outcomes, enhance healthcare delivery, and drive medical innovation. By leveraging AI-powered technologies and embracing a culture of innovation and collaboration, the healthcare industry can harness the full potential of AI to address current challenges and usher in a new era of personalized, efficient, and accessible healthcare for all.
Challenges of AI in Health
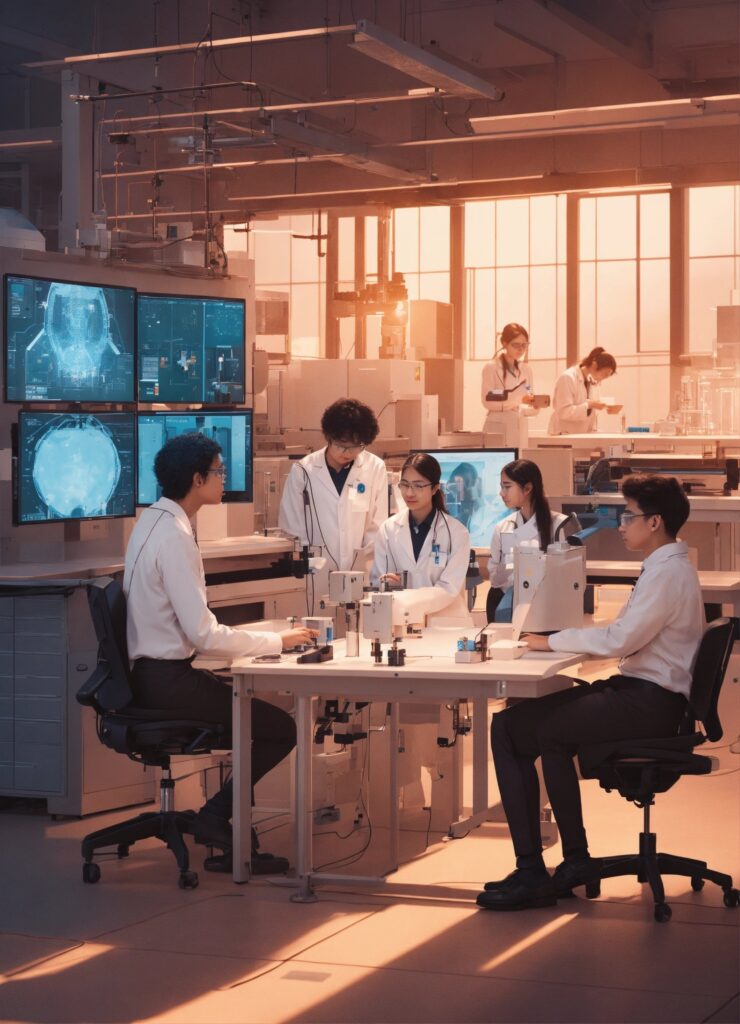
While artificial intelligence (AI) holds immense promise to revolutionize healthcare, its implementation also presents significant challenges that must be addressed to ensure its safe and effective integration into clinical practice. Here are some of the key challenges associated with AI in health:
- Data Privacy and Security Concerns: AI systems rely heavily on access to large volumes of sensitive patient data, including medical records, imaging scans, and genetic information. Ensuring the privacy and security of this data is paramount to protect patient confidentiality and comply with regulatory requirements such as HIPAA (Health Insurance Portability and Accountability Act). However, AI algorithms are vulnerable to data breaches, hacking, and unauthorized access, raising concerns about patient privacy and data security.
- Bias and Fairness in AI Algorithms: AI algorithms can inadvertently perpetuate biases present in the data used to train them, leading to discriminatory outcomes and disparities in healthcare delivery. Biases related to race, gender, socioeconomic status, and geographic location can influence AI predictions and recommendations, exacerbating existing disparities in access and outcomes. Addressing bias in AI algorithms requires careful data curation, algorithmic transparency, and ongoing monitoring and evaluation to ensure fairness and equity in healthcare AI applications.
- Regulatory and Legal Challenges: The rapid pace of AI innovation in healthcare has outpaced regulatory frameworks and legal standards, creating uncertainty and ambiguity around AI governance and liability. Regulatory bodies struggle to keep pace with the evolving landscape of AI technologies, leading to inconsistent standards and guidelines for AI deployment in care. Moreover, liability issues related to AI errors, malfunctions, and adverse outcomes raise complex legal questions regarding accountability and responsibility, requiring clear policies and regulations to mitigate risks and protect patient rights.
- Integration and Adoption Issues: Integrating AI technologies into existing maintenance systems and workflows presents logistical and organizational challenges, including interoperability issues, user resistance, and workflow disruptions. Healthcare providers may lack the necessary training, resources, and incentives to effectively utilize AI tools in clinical practice, leading to low adoption rates and underutilization of AI capabilities. Overcoming these barriers requires investment in training and education, user-friendly interfaces, and organizational support structures to facilitate the seamless integration of AI into maintenance delivery.
- Ethical and Societal Implications: AI raises profound ethical and societal questions related to patient autonomy, consent, and the doctor-patient relationship. AI-driven decision-making processes may lack transparency and explainability, making it difficult for patients and clinicians to understand and trust AI-generated recommendations. Moreover, AI technologies have the potential to disrupt traditional roles and responsibilities in healthcare, raising concerns about job displacement, professional autonomy, and the ethical implications of AI-driven decision-making. Balancing the benefits of AI innovation with ethical considerations requires careful consideration of values, principles, and societal norms to ensure that AI serves the best interests of patients and upholds ethical standards in healthcare practice.
Overall, addressing the challenges of AI in maintenance requires a multi-faceted approach that encompasses technical, regulatory, ethical, and societal dimensions. By actively engaging stakeholders, fostering collaboration, and prioritizing patient-centric principles, the healthcare industry can harness the transformative potential of AI while mitigating risks and ensuring responsible AI deployment in healthcare.
Ethical Considerations of AI in Health
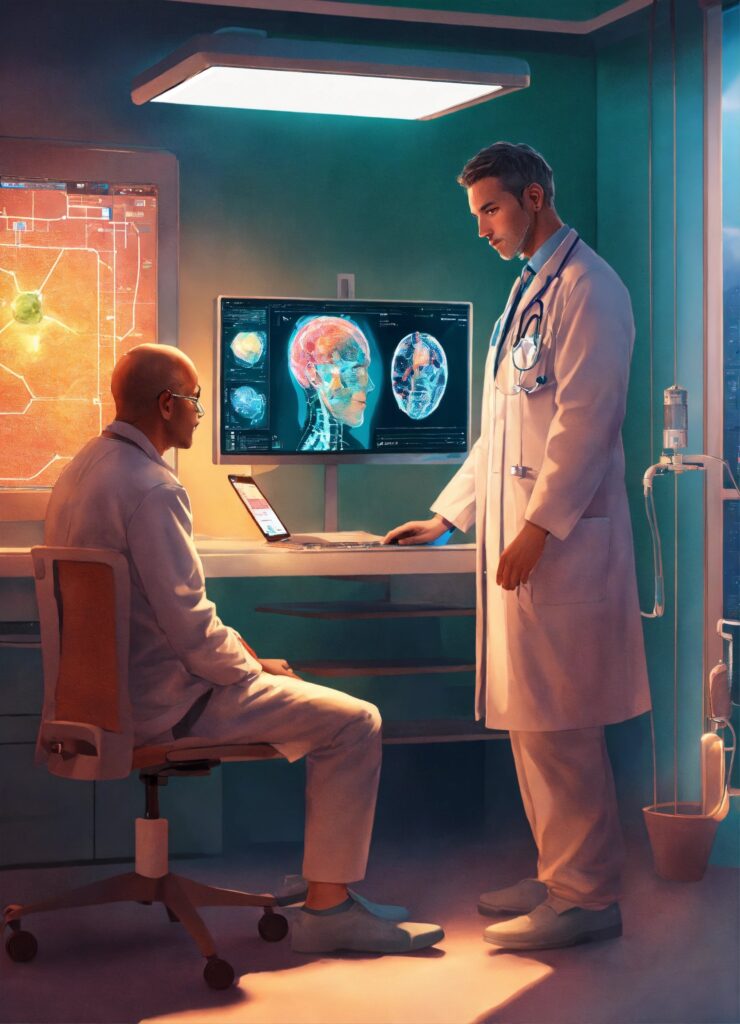
As artificial intelligence (AI) continues to advance in maintenance, it brings forth a multitude of ethical considerations that must be carefully navigated to ensure the responsible and ethical use of AI technologies. These ethical considerations encompass various aspects of AI deployment in healthcare, including patient autonomy, transparency, fairness, and accountability. Here are some of the key ethical considerations associated with AI in maintenance:
- Patient Autonomy and Informed Consent: AI-driven maintenance interventions may impact patient autonomy by influencing treatment decisions, diagnosis, and healthcare outcomes. Patients have the right to be informed about the use of AI in their care, including its potential benefits, risks, and limitations. Ensuring informed consent requires transparent communication, meaningful patient engagement, and respect for patient preferences and values.
- Transparency and Explainability: AI algorithms often operate as “black boxes,” making it challenging to understand the reasoning behind AI-generated decisions and recommendations. Lack of transparency and explainability can erode trust in AI systems and undermine patient and clinician confidence. Ethical AI design mandates transparency in AI algorithms, providing clear explanations of how decisions are made and enabling users to understand, validate, and interpret AI-generated outputs.
- Equity and Fairness: AI algorithms may perpetuate biases present in the data used to train them, leading to unfair or discriminatory outcomes, particularly for marginalized and vulnerable populations. Ensuring fairness and equity in AI-driven maintenance interventions requires careful data curation, algorithmic auditing, and mitigation strategies to address bias and promote inclusivity. Ethical AI design should prioritize fairness, equity, and social justice principles to mitigate disparities in maintenance access and outcomes.
- Data Privacy and Security: AI relies on access to large volumes of sensitive patient data, raising concerns about data privacy, confidentiality, and security. Protecting patient privacy requires robust data encryption, access controls, and compliance with privacy regulations such as HIPAA (Health Insurance Portability and Accountability Act). Ethical AI practices mandate the responsible handling of patient data, safeguarding against unauthorized access, breaches, and misuse.
- Accountability and Responsibility: AI-driven maintenance interventions raise questions of accountability and responsibility regarding errors, malfunctions, and adverse outcomes. Determining liability in AI-related incidents is complex and requires clear delineation of roles and responsibilities among AI developers, maintenance providers, and regulatory bodies. Ethical AI governance frameworks should establish mechanisms for accountability, risk management, and redress in the event of AI-related harm or wrongdoing.
- Professional Integrity and Autonomy: AI technologies have the potential to augment or replace certain aspects of clinical decision-making, raising questions about professional autonomy and integrity. maintenance professionals must maintain their ethical duty to act in the best interests of patients, critically evaluate AI-generated recommendations, and exercise clinical judgment in AI-assisted care. Ethical guidelines should uphold the primacy of the patient-provider relationship and ensure that AI complements, rather than supplants, clinical expertise and judgment.
Navigating the ethical considerations of AI in maintenance requires a collaborative and multidisciplinary approach that engages stakeholders across healthcare, technology, ethics, and policy domains. By upholding principles of transparency, fairness, accountability, and patient-centered care, the healthcare industry can harness the transformative potential of AI while ensuring that its deployment aligns with ethical values and promotes the well-being of patients and communities.
Case Studies and Examples
Examining real-world case studies and examples of artificial intelligence (AI) applications in maintenance provides valuable insights into the opportunities, challenges, and ethical considerations associated with AI deployment. Here are some illustrative case studies and examples that demonstrate the diverse applications of AI in maintenance:
- IBM Watson for Oncology:
- Case Study: IBM Watson for Oncology is an AI-powered platform designed to assist oncologists in treatment decision-making by analyzing patient data, medical literature, and expert guidelines to provide personalized treatment recommendations.
- Example: Memorial Sloan Kettering Cancer Center partnered with IBM to implement Watson for Oncology in clinical practice. However, a study published in JAMA Oncology found that Watson for Oncology provided discordant treatment recommendations compared to expert oncologists in certain cases, highlighting the importance of algorithm validation and clinical validation.
- DeepMind Health’s Streams:
- Case Study: DeepMind Health’s Streams is a mobile app that uses AI algorithms to analyze patient data and detect acute kidney injury (AKI) in hospitalized patients. Streams provides real-time alerts to clinicians, enabling early intervention and improved patient outcomes.
- Example: In a pilot study conducted at the Royal Free Hospital in London, Streams helped clinicians detect AKI cases significantly earlier than traditional methods, leading to reduced patient deterioration and hospital mortality rates. However, the partnership between DeepMind Health and the Royal Free Hospital raised concerns about patient data privacy and consent, prompting scrutiny from regulatory authorities and ethics committees.
- Google’s Medical Imaging AI:
- Case Study: Google has developed AI algorithms for medical imaging analysis, including the detection of diabetic retinopathy from retinal images and the identification of breast cancer from mammograms.
- Example: In a study published in Nature Medicine, Google’s AI model demonstrated high accuracy in detecting breast cancer from mammograms, outperforming radiologists in certain cases. However, concerns have been raised about the potential for AI algorithms to exacerbate existing disparities in healthcare access and outcomes, particularly if they are not validated across diverse patient populations.
- Telemedicine Platforms:
- Case Study: Telemedicine platforms leverage AI technologies to facilitate virtual consultations, remote diagnosis, and remote patient monitoring, enabling patients to access healthcare services remotely.
- Example: During the COVID-19 pandemic, telemedicine platforms experienced a surge in adoption as healthcare providers sought alternative ways to deliver care while minimizing in-person contact. Platforms such as Teladoc, Amwell, and Doctor on Demand use AI-driven triage algorithms to prioritize patient cases and optimize healthcare delivery.
- Chatbots and Virtual Assistants:
- Case Study: Chatbots and virtual assistants powered by AI algorithms provide patients with personalized health information, symptom assessment, and triage recommendations.
- Example: Babylon Health’s chatbot, powered by natural language processing (NLP) algorithms, offers symptom checking and health advice to users via a mobile app. While chatbots have the potential to improve healthcare access and patient engagement, concerns have been raised about the accuracy of symptom assessment and the need for human oversight to ensure patient safety.
These case studies and examples illustrate the diverse applications of AI in healthcare, ranging from diagnostic support and treatment decision-making to patient monitoring and telemedicine. While AI holds immense promise to improve healthcare outcomes and efficiency, careful consideration of regulatory, ethical, and societal implications is essential to ensure responsible AI deployment and mitigate potential risks. By learning from both successes and challenges in AI implementation, the healthcare industry can harness the full potential of AI while upholding ethical principles and promoting patient-centric care.
Conclusion
The integration of artificial intelligence (AI) into healthcare holds transformative potential to revolutionize medical practice, improve patient outcomes, and enhance healthcare delivery. Throughout this essay, we have explored the myriad opportunities, challenges, and ethical considerations associated with AI deployment in health. AI presents opportunities to advance diagnosis and disease detection through sophisticated algorithms that analyze medical images, genomic data, and clinical records with unparalleled accuracy and efficiency. Personalized treatment and precision medicine approaches powered by AI offer the promise of tailoring interventions to individual patient profiles, maximizing efficacy while minimizing adverse effects.
Moreover, AI has the potential to enhance healthcare delivery by streamlining administrative tasks, optimizing resource allocation, and facilitating remote monitoring and telemedicine. By harnessing the power of predictive analytics and machine learning, healthcare providers can improve operational efficiency, reduce wait times, and enhance the overall patient experience. However, the widespread adoption of AI in health also presents significant challenges and ethical considerations that must be carefully addressed. Concerns about data privacy and security, algorithmic bias, regulatory oversight, and accountability loom large, highlighting the need for robust governance frameworks and ethical guidelines to safeguard patient rights and ensure responsible AI deployment.
In navigating the complex landscape of AI in health, it is imperative to uphold ethical principles of transparency, fairness, equity, and patient-centered care. By fostering interdisciplinary collaboration, promoting stakeholder engagement, and prioritizing patient well-being, the care industry can harness the transformative potential of AI while mitigating risks and addressing societal concerns. For more information you can check our blogs Finding Equilibrium: Navigating Screen Time for Vibrant Eye Health.